Topic Menu
► Topic MenuTopic Editors



Artificial Intelligence and Sustainable Energy Systems
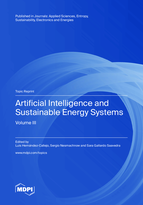
A printed edition is available here.
Topic Information
Dear Colleagues,
The problems that affect humanity are numerous and occur in different areas. Energy sustainability, climate change and the effects derived from pollutants and viruses are some of the most relevant problems. The main objective of researchers is to provide solutions to these and other problems.
In recent years, the use of artificial intelligence has increased considerably. Artificial intelligence is used in different areas: energy, sustainability, medicine, health, mobility, industry, etc. Therefore, it is necessary to continue advancing in the application of artificial intelligence to the aforementioned problems. Energy is a precious commodity, and it is increasingly difficult to dispose of energy in a sustainable way. In this sense, renewable energy sources are essential, although the use of conventional energy cannot be forgotten. Therefore, sustainable energy systems, integrating renewable and non-renewable energy sources, smart systems and new business models, are crucial.
Hence, contributions are expected to involve affect artificial intelligence (in any area) and the development and evolution of sustainable energy systems.
Prof. Dr. Luis Hernández-Callejo
Dr. Sergio Nesmachnow
Dr. Sara Gallardo Saavedra
Topic Editors
Keywords
- artificial intelligence
- expert systems
- machine learning
- deep learning
- modelling
- prediction
- probabilistic models
- prediction models
- sustainable mobility
- smart cities
- smart grids
- sustainable energy systems
- renewable energy sources
- nonrenewable energy sources
- electrical storage
- green hydrogen
- sustainable greenhouses
- wind
- microgrids
- tidal
- solar
- biomass
- power network
- solar thermal
- solar photovoltaic
- hydraulics
- ANN
- neural networks, fuzzy logic, genetic algorithms
- hybrid models based on artificial intelligence
Participating Journals
Journal Name | Impact Factor | CiteScore | Launched Year | First Decision (median) | APC |
---|---|---|---|---|---|
![]()
Applied Sciences
|
2.7 | 4.5 | 2011 | 16.9 Days | CHF 2400 |
![]()
Entropy
|
2.7 | 4.7 | 1999 | 20.8 Days | CHF 2600 |
![]()
Sustainability
|
3.9 | 5.8 | 2009 | 18.8 Days | CHF 2400 |
![]()
Electronics
|
2.9 | 4.7 | 2012 | 15.6 Days | CHF 2400 |
![]()
Energies
|
3.2 | 5.5 | 2008 | 16.1 Days | CHF 2600 |
MDPI Topics is cooperating with Preprints.org and has built a direct connection between MDPI journals and Preprints.org. Authors are encouraged to enjoy the benefits by posting a preprint at Preprints.org prior to publication:
- Immediately share your ideas ahead of publication and establish your research priority;
- Protect your idea from being stolen with this time-stamped preprint article;
- Enhance the exposure and impact of your research;
- Receive feedback from your peers in advance;
- Have it indexed in Web of Science (Preprint Citation Index), Google Scholar, Crossref, SHARE, PrePubMed, Scilit and Europe PMC.