Topic Editors

2. Electrical and Computer Engineering, University of Western Macedonia, 5010 Kozani, Greece
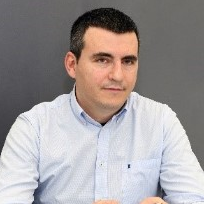



Next Generation Intelligent Communications and Networks
Topic Information
Dear Colleagues,
As a response to the spectrum scarcity caused by the aggressive proliferation of wireless devices and quality-of-service (QoS) and quality-of-experience (QoE) hungry services, which are expected to support a broad range of diverse multi-scale and multi-environment applications, sixth-generation (6G) wireless networks have adopted higher frequency bands, such as the millimenter wave, the terahertz (THz) and the optical band. High-frequency wireless communications are recognized as a technological enabler of a varied set of use cases, from in-body nano-scale networks to indoor and outdoor wireless personal/local area and fronthaul/backhaul networks. Nano-scale applications require compact transceiver designs and self-organized ad hoc network topologies. On the other hand, macro-scale applications demand flexibility, sustainability, adaptability and security in an ever-changing heterogeneous environment. Moreover, the ability to support a high data rate of up to 1 Tb/s and energy-efficient massive connectivity are only some of the key demands. To address the aforementioned requirements, artificial intelligence (AI), in combination with novel structures capable of altering the wireless environment, have been regarded as complementary pillars to 6G wireless THz systems. AI is expected to enable a series of new features in next-generation networks, including, but not limited to: self-aggregation, context awareness, self-configuration and opportunistic deployment. In addition, integrating AI in wireless networks is predicted to bring about a revolutionary transformation of conventional cognitive radio systems into intelligent platforms by unlocking the full potential of radio signals and exploiting new degrees of freedom. Considering this context, this Special Issue aims to present papers investigating AI-empowered and/or -enabled next-generation wireless systems and networks. Potential topics include, but are not limited to, the following:
- Identification of communication systems and networks requirements that call for the use of AI approaches.
- AI-enabled architectures with an emphasis on open radio-access networks, SD fabric and verticals, such as agriculture, self-driving vehicles, automation, industry 4.0, etc.
- Semantic and task-oriented communications beyond Shannon performance.
- Topics related to AI-empowered physical layers, such as: machine learning channel modeling and/or estimation approaches based on point cloud ray tracing algorithms or similar schemes, as well as channel prediction, involving reconfigurable-intelligent-surface-enabled wireless systems; modulation recognition and signal detection in complex wireless environments; and analog, digital, hybrid and reconfigurable intelligent surface (RIS) beamforming design.
- Medium and multiple access control: 3D radio resource management, channel allocation, power management, blockage avoidance schemes, localization approaches, pro-active and predictive mobility management, intelligent routing, etc.
- Novel AI deployment schemes for next-generation networks.
Dr. Alexandros-Apostolos Boulogeorgos
Dr. Panagiotis Sarigiannidis
Dr. Thomas Lagkas
Prof. Dr. Vasileios Argyriou
Prof. Dr. Pantelis Angelidis
Topic Editors
Keywords
- artificial intelligence
- explainable AI
- federated learning
- machine learning
- medium and multiple access control
- physical layer
- radio resource management
- reinforcement learning
- transfer learning
- semantic communications
Participating Journals
Journal Name | Impact Factor | CiteScore | Launched Year | First Decision (median) | APC | |
---|---|---|---|---|---|---|
![]()
Applied Sciences
|
2.7 | 4.5 | 2011 | 16.9 Days | CHF 2400 | Submit |
![]()
Digital
|
- | - | 2021 | 22.7 Days | CHF 1000 | Submit |
![]()
Electronics
|
2.9 | 4.7 | 2012 | 15.6 Days | CHF 2400 | Submit |
![]()
Sensors
|
3.9 | 6.8 | 2001 | 17 Days | CHF 2600 | Submit |
![]()
Telecom
|
- | 3.1 | 2020 | 26.1 Days | CHF 1200 | Submit |
MDPI Topics is cooperating with Preprints.org and has built a direct connection between MDPI journals and Preprints.org. Authors are encouraged to enjoy the benefits by posting a preprint at Preprints.org prior to publication:
- Immediately share your ideas ahead of publication and establish your research priority;
- Protect your idea from being stolen with this time-stamped preprint article;
- Enhance the exposure and impact of your research;
- Receive feedback from your peers in advance;
- Have it indexed in Web of Science (Preprint Citation Index), Google Scholar, Crossref, SHARE, PrePubMed, Scilit and Europe PMC.