Applied Computer Vision in Industry and Agriculture
A special issue of Applied Sciences (ISSN 2076-3417). This special issue belongs to the section "Computing and Artificial Intelligence".
Deadline for manuscript submissions: 30 June 2024 | Viewed by 6553
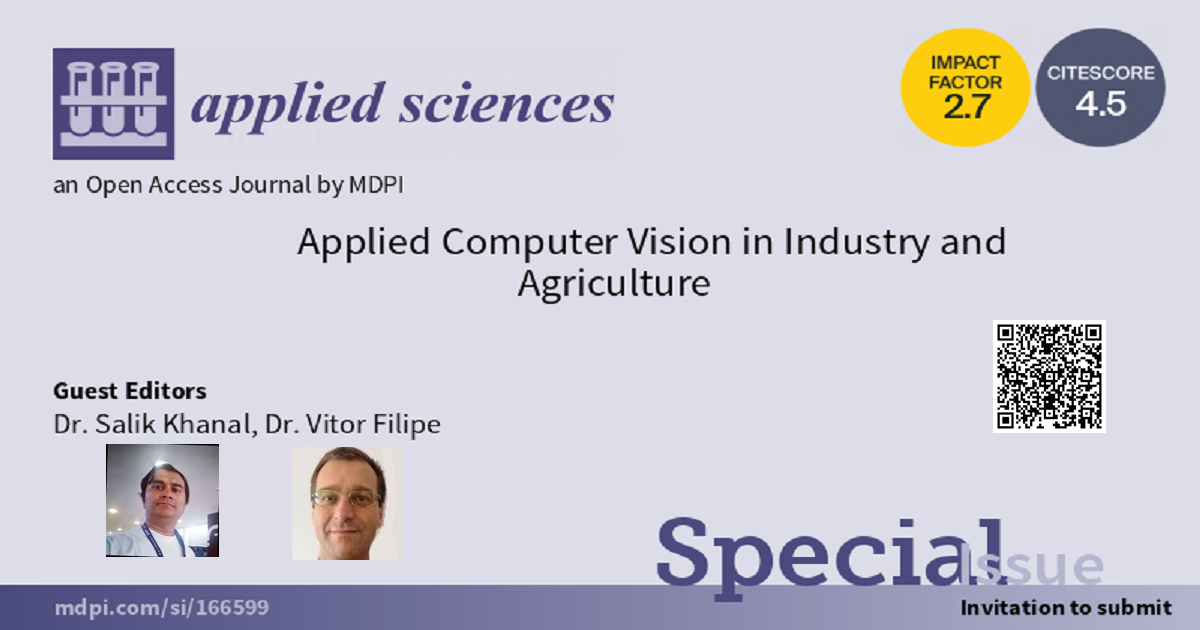
Special Issue Editors
Interests: machine vision; field robotics; computer vision; machine learning; Industry Technology 4.0
Special Issues, Collections and Topics in MDPI journals
Interests: computer vision; machine learning; animal and human movement analysis
Special Issues, Collections and Topics in MDPI journals
Special Issue Information
Dear Colleagues,
In recent years, machine vision has been applied in a wide range of areas, including industry, agriculture, psychology, sports, etc. With the advancement of computer vision technology, industrial technology is moving into another era, which has led to an improvement in the overall gain. This Special Issue aims to publish high-quality articles that represent cutting-edge research on the development of machine vision-based industry and agriculture automation. This Special Issue also aims to promote and motivate research to obtain better technology using computer vision in industry and agriculture automation.
The topics include, but are not limited to:
- Machine vision in industry and agriculture;
- Industrial automation;
- Industrial robotics;
- Agriculture automation using machine vision;
- Anomaly detection using machine vision;
- Industrial robot design;
- 3D vision technology in industry and agriculture;
- Applied computer science in industry.
Dr. Salik Ram Khanal
Dr. Vitor Filipe
Guest Editors
Manuscript Submission Information
Manuscripts should be submitted online at www.mdpi.com by registering and logging in to this website. Once you are registered, click here to go to the submission form. Manuscripts can be submitted until the deadline. All submissions that pass pre-check are peer-reviewed. Accepted papers will be published continuously in the journal (as soon as accepted) and will be listed together on the special issue website. Research articles, review articles as well as short communications are invited. For planned papers, a title and short abstract (about 100 words) can be sent to the Editorial Office for announcement on this website.
Submitted manuscripts should not have been published previously, nor be under consideration for publication elsewhere (except conference proceedings papers). All manuscripts are thoroughly refereed through a single-blind peer-review process. A guide for authors and other relevant information for submission of manuscripts is available on the Instructions for Authors page. Applied Sciences is an international peer-reviewed open access semimonthly journal published by MDPI.
Please visit the Instructions for Authors page before submitting a manuscript. The Article Processing Charge (APC) for publication in this open access journal is 2400 CHF (Swiss Francs). Submitted papers should be well formatted and use good English. Authors may use MDPI's English editing service prior to publication or during author revisions.
Keywords
- machine vision
- computer vision
- agriculture automation
- industry automation