Deep Learning for Image Recognition and Processing
A special issue of Applied Sciences (ISSN 2076-3417). This special issue belongs to the section "Computing and Artificial Intelligence".
Deadline for manuscript submissions: 20 July 2024 | Viewed by 6652
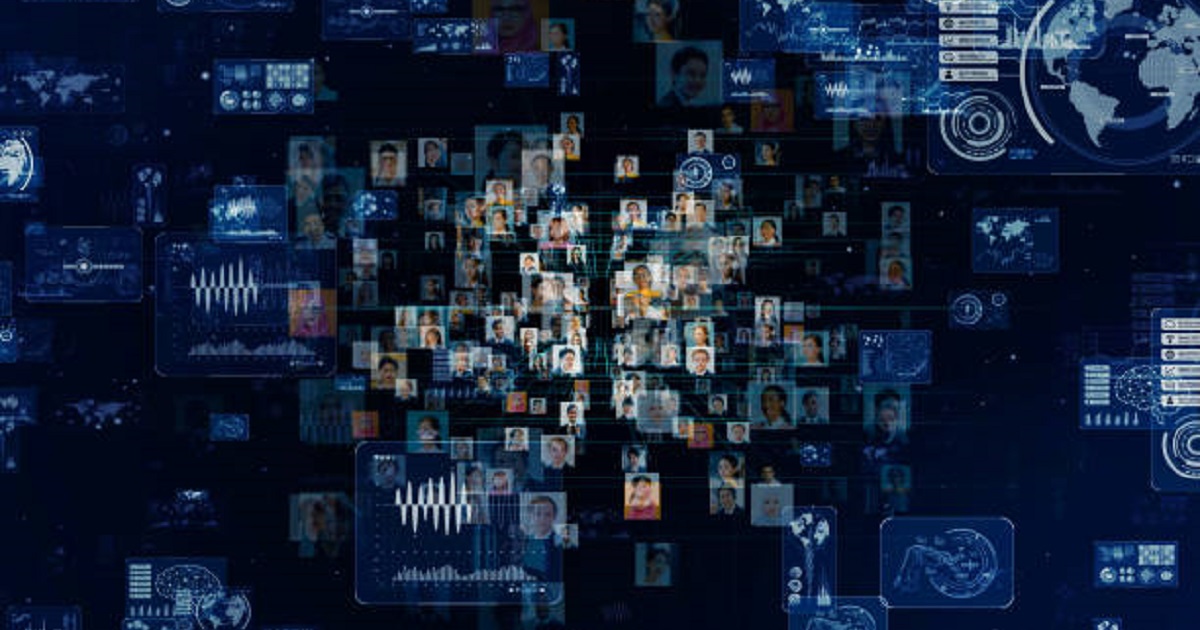
Special Issue Editors
Interests: image and video semantic segmentation; deep learning; industrial process control; industrial intelligence; natural language processing; knowledge graph
Special Issues, Collections and Topics in MDPI journals
Interests: machine learning; deep learning; remote sensing image analysis; image segmentation
Special Issue Information
Dear Colleagues,
Deep learning technology has been drawing increasing interest for a wide range of computer vision and image analysis tasks, such as image classification, image segmentation, object detection and so on. A number of applications can be utilized by deep learning technology, such as industrial intelligence, remote sensing image analysis and autonomous driving. However, deep learning technology has faced some challenging problems in various applications, limiting image recognition and processing performance. Some modified deep learning on model-based or module-based strategies cannot cope with the volume of problems, and this must be urgently addressed: for example, how to improve deep learning model accuracy by using a limited sample size, or how to propose an explainable deep learning model to make it precise and reliable. As a result, this Special Issue aims to cover novel strategies on deep learning algorithms for image recognition and processing by using reliable, optimized and hybrid deep learning algorithms in a number of applications.
In this Special Issue, original research articles and reviews are welcome. Research areas may include (but are not limited to) the following:
- Deep learning image analysis in civil applications (e.g., industrial intelligence, remote sensing, biology and medical image).
- Novel training strategies when facing small sample size problems.
- Explainable deep learning models to innovate deep learning model construction and improve model reliability.
- Image classification, segmentation and object targeting using deep learning algorithms.
- The transfer of learning and knowledge distillation for image processing.
We look forward to receiving your contributions.
Prof. Dr. Jiangyun Li
Dr. Tianxiang Zhang
Dr. Peixian Zhuang
Guest Editors
Manuscript Submission Information
Manuscripts should be submitted online at www.mdpi.com by registering and logging in to this website. Once you are registered, click here to go to the submission form. Manuscripts can be submitted until the deadline. All submissions that pass pre-check are peer-reviewed. Accepted papers will be published continuously in the journal (as soon as accepted) and will be listed together on the special issue website. Research articles, review articles as well as short communications are invited. For planned papers, a title and short abstract (about 100 words) can be sent to the Editorial Office for announcement on this website.
Submitted manuscripts should not have been published previously, nor be under consideration for publication elsewhere (except conference proceedings papers). All manuscripts are thoroughly refereed through a single-blind peer-review process. A guide for authors and other relevant information for submission of manuscripts is available on the Instructions for Authors page. Applied Sciences is an international peer-reviewed open access semimonthly journal published by MDPI.
Please visit the Instructions for Authors page before submitting a manuscript. The Article Processing Charge (APC) for publication in this open access journal is 2400 CHF (Swiss Francs). Submitted papers should be well formatted and use good English. Authors may use MDPI's English editing service prior to publication or during author revisions.
Keywords
- machine learning/deep learning
- image segmentation
- image classification
- object detection
- remote sensing image analysis