AI Technologies for Collaborative and Service Robots
A special issue of Applied Sciences (ISSN 2076-3417). This special issue belongs to the section "Robotics and Automation".
Deadline for manuscript submissions: 20 June 2024 | Viewed by 2122
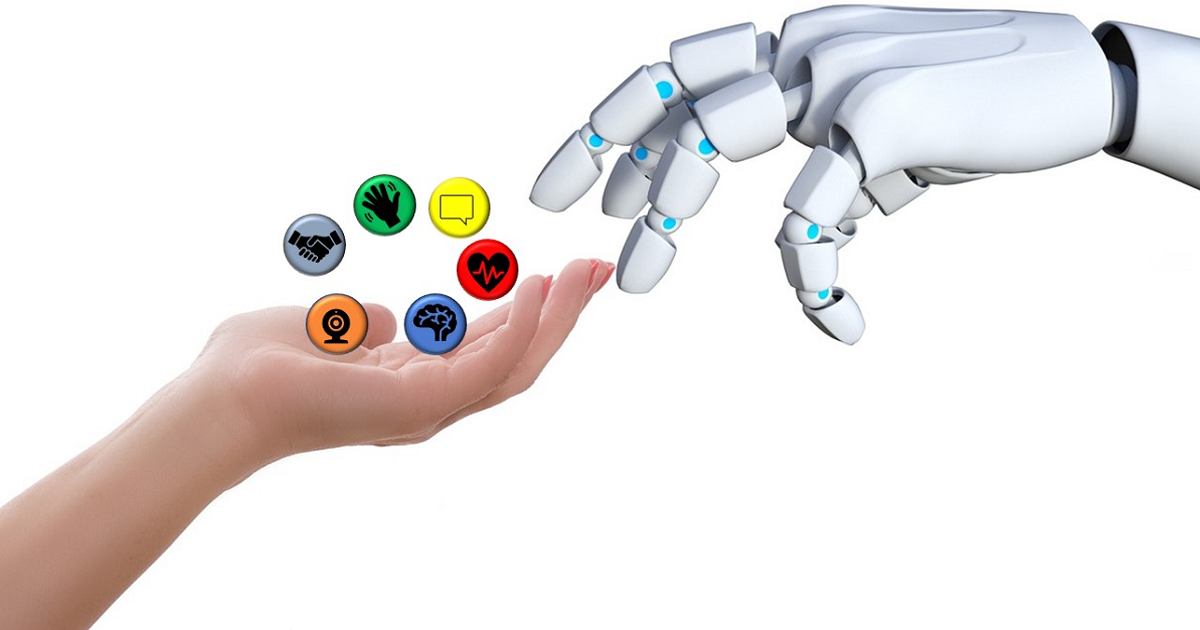
Special Issue Editors
Interests: industrial and collaborative robotics; robot and mechanism design; performance evaluation; cable-driven robots
Special Issues, Collections and Topics in MDPI journals
Interests: robotics; redundant robots; trajectory optimization; collaborative robots; rehabilitative robotics; dynamic models
Special Issues, Collections and Topics in MDPI journals
Special Issue Information
Dear Colleagues,
Nowadays, new technologies are improving safe human-robot interactions, and robots are becoming increasingly common both inside and outside of industrial scenarios. Not only are collaborative robots improving production plants by shifting the paradigm of industrial robotics from servant devices to intelligent coworkers, but also service, surgical, and rehabilitation robotics are becoming more and more significant, both from a technological and economic point of view, but also for their social implications. In this context, new intelligent technologies are required for controlling robots in unpredictable and cluttered environments. Moreover, for collaborative and service robots to be effective, they need to become more intelligent, adaptable, and capable of predicting human behaviors in human-centered environments.
For these reasons, we are pleased to invite you to submit a paper for a Special Issue titled “AI Technologies for Collaborative and Service Robots”. This Special Issue aims to provide an opportunity for researchers to publish technological studies and advancements addressing the design and development of applications for collaborative and service robots.
This Special Issue will publish high-quality, original research papers that are related to theory, design, practice, and applications of collaborative and service robots, including, but not limited to, fields of:
- Indoor localization and navigation;
- Control of robotic systems;
- Innovative robots and applications;
- Service, medical, and assistive robotics;
- Haptics;
- Artificial Intelligence;
- Machine learning approaches;
- Dynamics of robots and multi-body systems;
- Human-robot communication;
- Autonomous mobile robotics.
Prof. Dr. Giovanni Boschetti
Dr. Matteo Bottin
Dr. Riccardo Minto
Guest Editors
Manuscript Submission Information
Manuscripts should be submitted online at www.mdpi.com by registering and logging in to this website. Once you are registered, click here to go to the submission form. Manuscripts can be submitted until the deadline. All submissions that pass pre-check are peer-reviewed. Accepted papers will be published continuously in the journal (as soon as accepted) and will be listed together on the special issue website. Research articles, review articles as well as short communications are invited. For planned papers, a title and short abstract (about 100 words) can be sent to the Editorial Office for announcement on this website.
Submitted manuscripts should not have been published previously, nor be under consideration for publication elsewhere (except conference proceedings papers). All manuscripts are thoroughly refereed through a single-blind peer-review process. A guide for authors and other relevant information for submission of manuscripts is available on the Instructions for Authors page. Applied Sciences is an international peer-reviewed open access semimonthly journal published by MDPI.
Please visit the Instructions for Authors page before submitting a manuscript. The Article Processing Charge (APC) for publication in this open access journal is 2400 CHF (Swiss Francs). Submitted papers should be well formatted and use good English. Authors may use MDPI's English editing service prior to publication or during author revisions.
Keywords
- collaborative robots
- human-robot interaction
- autonomous service robots
- virtual assistants
- artificial intelligence, machine learning and deep learning
- prediction models
- augmented and virtual reality