Intelligent Manufacturing and Medical-Engineering Integration
A special issue of Applied Sciences (ISSN 2076-3417). This special issue belongs to the section "Mechanical Engineering".
Deadline for manuscript submissions: 30 November 2024 | Viewed by 1985
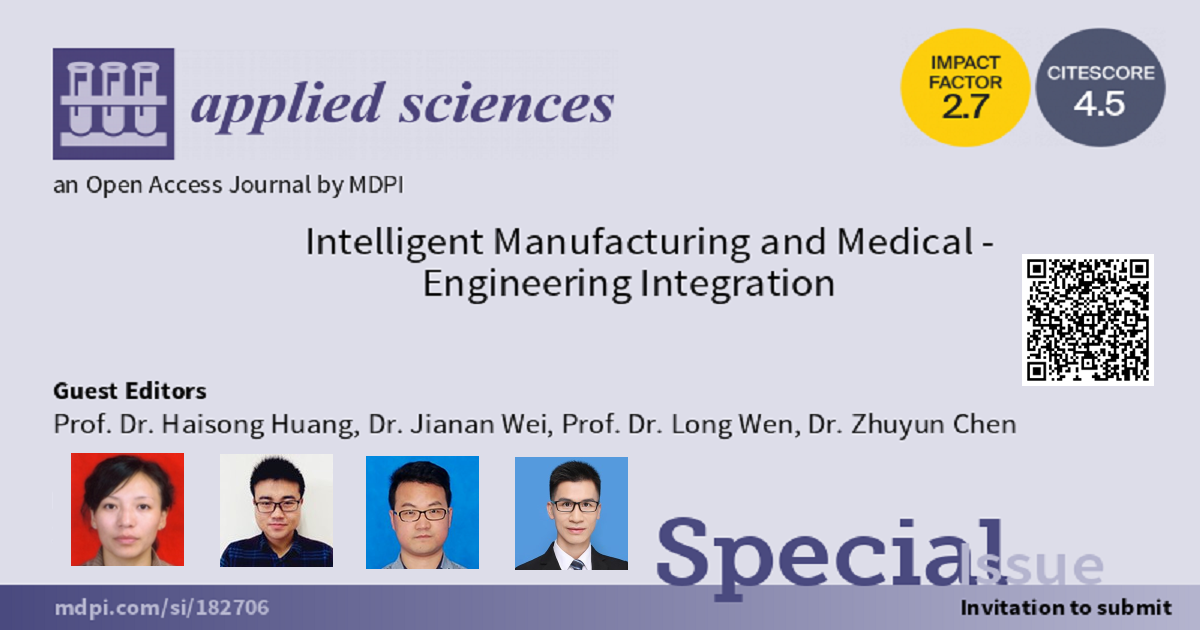
Special Issue Editors
Interests: intelligent manufacturing; manufacturing big data and manufacturing information systems
Special Issues, Collections and Topics in MDPI journals
Interests: manufacturing big data and manufacturing information systems; intelligent manufacturing; machine learning; deep transfer learning; fault diagnosis; imbalanced data processing and predictive maintenance
Special Issues, Collections and Topics in MDPI journals
Interests: deep learning; automatic machine learning; fault diagnosis; intelligent algorithm
Special Issues, Collections and Topics in MDPI journals
Interests: deep transfer learning; federated learning; signal processing; fault diagnosis
Special Issues, Collections and Topics in MDPI journals
Special Issue Information
Dear Colleagues,
This Special Issue aims to explore research and applications in cutting-edge fields such as Manufacturing Big Data and Information Systems, Intelligent Manufacturing and Maintenance, and Medical-Engineering Integration Manufacturing. With the intelligent transformation of the manufacturing industry, big data technology is playing an increasingly important role in production processes. This Special Issue will focus on how to apply advanced technologies like big data analytics, machine learning, and deep learning to achieve process optimization, fault prediction and maintenance, and resource utilization maximization in manufacturing. Additionally, this Special Issue will also address the cross-disciplinary domains of intelligent manufacturing and medical-engineering integration, investigating the application of intelligent manufacturing technologies in the manufacturing and operation of medical devices to enhance the efficiency and quality of the medical industry. Scholars from academia and industry are welcome to participate and share the latest research findings and innovative ideas in the fields of intelligent manufacturing and medical-engineering integration.
Potential topics include, but are not limited to:
- Intelligent fault prediction and prevention driven by big data: Utilizing big data analysis and machine learning to achieve early prediction and prevention of equipment failures, optimizing production processes, and reducing maintenance costs.
- Intelligent fault diagnosis models and algorithms: Researching algorithms like imbalanced learning, few-shot learning, positive-unlabeled learning (PU learning), zero-shot learning, and modeling methods under various operating conditions to provide efficient and accurate solutions for mechanical equipment fault diagnosis.
- Cross-disciplinary innovation in medical-engineering integration manufacturing: Applying intelligent manufacturing technology to the manufacturing and operation of medical devices to improve the efficiency and quality of the medical industry.
- Advancements in fault diagnosis/prediction and remaining useful life estimation using deep learning algorithms: Exploring the application of deep learning in data analysis and remaining useful life prediction for achieving more accurate predictive maintenance.
- Data-driven medical diagnosis: Utilizing deep learning and data analysis techniques for intelligent diagnosis of medical images and patient data to enhance the accuracy and efficiency of medical diagnosis.
- Construction and optimization of manufacturing information systems: Building intelligent manufacturing information systems and incorporating technologies like imbalanced data processing, time series data analysis, and natural language processing to optimize manufacturing processes, improving efficiency and quality in manufacturing.
Prof. Dr. Haisong Huang
Dr. Jianan Wei
Prof. Dr. Long Wen
Dr. Zhuyun Chen
Guest Editors
Manuscript Submission Information
Manuscripts should be submitted online at www.mdpi.com by registering and logging in to this website. Once you are registered, click here to go to the submission form. Manuscripts can be submitted until the deadline. All submissions that pass pre-check are peer-reviewed. Accepted papers will be published continuously in the journal (as soon as accepted) and will be listed together on the special issue website. Research articles, review articles as well as short communications are invited. For planned papers, a title and short abstract (about 100 words) can be sent to the Editorial Office for announcement on this website.
Submitted manuscripts should not have been published previously, nor be under consideration for publication elsewhere (except conference proceedings papers). All manuscripts are thoroughly refereed through a single-blind peer-review process. A guide for authors and other relevant information for submission of manuscripts is available on the Instructions for Authors page. Applied Sciences is an international peer-reviewed open access semimonthly journal published by MDPI.
Please visit the Instructions for Authors page before submitting a manuscript. The Article Processing Charge (APC) for publication in this open access journal is 2400 CHF (Swiss Francs). Submitted papers should be well formatted and use good English. Authors may use MDPI's English editing service prior to publication or during author revisions.
Keywords
- intelligent manufacturing
- medical-engineering integration manufacturing
- intelligent maintenance
- manufacturing big data
Related Special Issue
- Mechatronics System Design in Medical Engineering in Applied Sciences (5 articles)