-
Evaluating the Performance of Automated Machine Learning (AutoML) Tools for Heart Disease Diagnosis and Prediction
-
Chat GPT in Diagnostic Human Pathology: Will It Be Useful to Pathologists? A Preliminary Review with ‘Query Session’ and Future Perspectives
-
A Comprehensive Review of AI Techniques for Addressing Algorithmic Bias in Job Hiring
Journal Description
AI
- Open Access— free for readers, with article processing charges (APC) paid by authors or their institutions.
- High Visibility: indexed within ESCI (Web of Science), Scopus, EBSCO, and other databases.
- Rapid Publication: manuscripts are peer-reviewed and a first decision is provided to authors approximately 20.8 days after submission; acceptance to publication is undertaken in 5.8 days (median values for papers published in this journal in the second half of 2023).
- Recognition of Reviewers: APC discount vouchers, optional signed peer review, and reviewer names published annually in the journal.
Latest Articles
Highly Accessed Articles
Latest Books
E-Mail Alert
News
Meet Us at the ISPRS TC I Mid-term Symposium on Intelligent Sensing and Remote Sensing Application (ISPRS 2024 TC I), 13–17 May 2024, Changsha, China
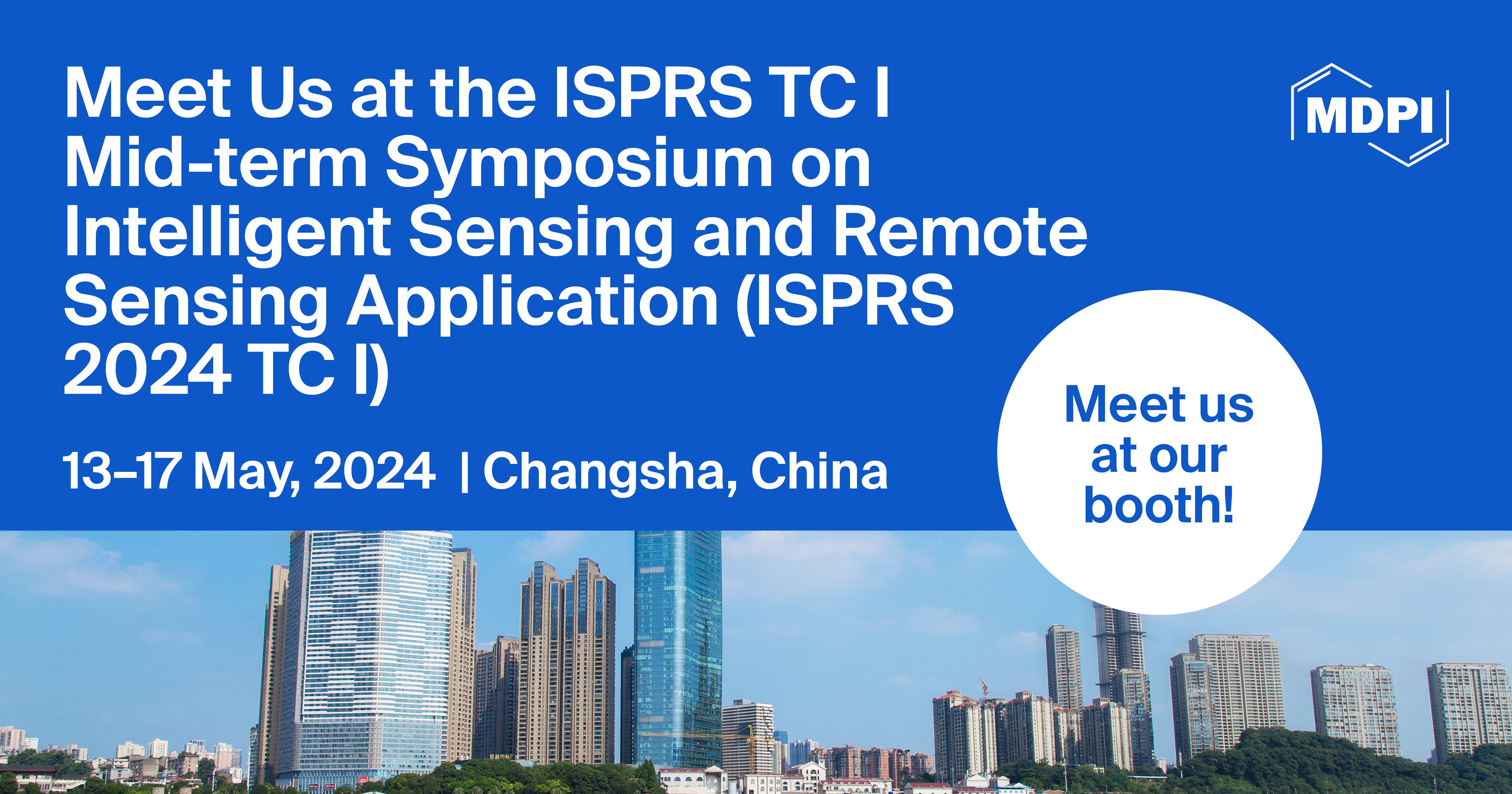
Topics
Deadline: 30 April 2024
Deadline: 31 May 2024
Deadline: 30 June 2024
Deadline: 8 August 2024
Conferences
Special Issues
Deadline: 30 June 2024
Deadline: 15 July 2024
Deadline: 31 July 2024
Deadline: 31 August 2024