Topic Menu
► Topic MenuTopic Editors

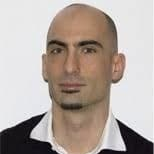

2. Faculty of Medicine, Department of Surgery & Cancer, Imperial College Faculty of Medicine, London SW7 2BX, UK

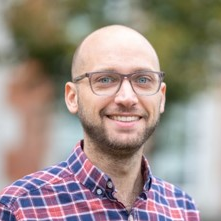
2. Lysholm Department of Neuroradiology, National Hospital for Neurology and Neurosurgery, University College London Hospitals NHS Foundation Trust, London WC1E 6BT, UK



Application of Machine Learning in Molecular Imaging
Topic Information
Dear Colleagues,
The term molecular imaging (MI) refers to an increasingly powerful set of techniques with the ability to identify and quantify in vivo a broad range of biologic processes both at the cellular and subcellular levels, spanning positron emission tomography (PET), single-photon-emission computed tomography (SPECT), magnetic resonance imaging (MRI) and spectroscopy (MRS), computed tomography (CT), ultrasound (US), and optical imaging (OI), among others.
All facets of MI—from image acquisition through diagnosis—have been impacted by artificial intelligence (AI) and machine learning (ML) to varying degrees. MI analysis has changed significantly over the past ten years, especially with the development of deep learning. Although neural-network models used for image segmentation have accounted for most recent developments, a wide range of techniques have shown promise for image reconstruction, image synthesis, differential diagnostic generation, and therapy recommendation. Signal-based receivers have greatly benefited from the use of, e.g., Rényi Entropy in probe design, and surface dissipation as well as entropy have been used to stabilize single-molecule reaction intermediates. Additionally, the operation of the interrelated parts of the immune system in both health and sickness can be rigorously mathematically described and formalized using information theory. On various temporal and spatial scales, one may measure the storage, transmission, encoding, and flow of information inside and between cell components of the immune system using concepts like entropy, mutual information, and channel capacity. The use of AI to facilitate molecular-probe design, which is still in its early stages, is being fueled by applications in drug design. In PET reconstruction from sinogram data, deep-learning models have shown improved productivity and image quality. Additional applications have been identified for specialized architectures like generative adversarial networks (GANs) in the creation of synthetic PET and CT images, modality transition, artifact elimination, ultralow-dose contrast-enhanced MRI imaging and radioisotopic imaging. Some AI systems that are partially or entirely based on neural network techniques have outperformed radiologists in differential diagnosis tasks. However, AI models have a reputation for being brittle, and clinicians as well as patients might not trust AI paradigms that are not able to articulate their logic. Most AI solutions for molecular imaging are currently still restricted to the research domain, while integration into standard clinical workflows through commercialization and, in some cases, hardware integration is in its infancy. In this respect, the assessment of AI’s utility in molecular imaging based on actual clinical products is direly needed.
This Topic aims to collect state-of-the-art, unpublished research contributions in the areas of supervised, unsupervised, and reinforcement-based machine learning and deep learning applied to PET, SPECT, MRI and MRS, CT, US and OI.
Topics of interest include but are not limited to:
- Molecular imaging hardware design;
- RF pulse design for MR imaging;
- Entropy- and information-theory-based strategies for molecular probe optimization;
- Image reconstruction, denoising, under- and oversampling;
- Statistical inference on molecular imaging data;
- Multimodal image integration;
- Specialized processing (e.g., image segmentation, lesion identification);
- Explainability strategies in image-based inference;
- Computer-assisted diagnosis and prognosis in molecular imaging;
- Ethical aspects of AI in molecular imaging.
Dr. Allegra Conti
Dr. Nicola Toschi
Dr. Marianna Inglese
Dr. Andrea Duggento
Dr. Matthew Grech-Sollars
Dr. Serena Monti
Dr. Giancarlo Sportelli
Dr. Pietro Carra
Topic Editors
Keywords
- machine learning
- deep learning
- molecular imaging
- nuclear medicine
- statistical inference on medical images
- image reconstruction
- AI-enhanced imaging hardware
Participating Journals
Journal Name | Impact Factor | CiteScore | Launched Year | First Decision (median) | APC | |
---|---|---|---|---|---|---|
![]()
Algorithms
|
2.3 | 3.7 | 2008 | 15 Days | CHF 1600 | Submit |
![]()
Diagnostics
|
3.6 | 3.6 | 2011 | 20.7 Days | CHF 2600 | Submit |
![]()
Entropy
|
2.7 | 4.7 | 1999 | 20.8 Days | CHF 2600 | Submit |
![]()
Information
|
3.1 | 5.8 | 2010 | 18 Days | CHF 1600 | Submit |
![]()
Journal of Imaging
|
3.2 | 4.4 | 2015 | 21.7 Days | CHF 1800 | Submit |
MDPI Topics is cooperating with Preprints.org and has built a direct connection between MDPI journals and Preprints.org. Authors are encouraged to enjoy the benefits by posting a preprint at Preprints.org prior to publication:
- Immediately share your ideas ahead of publication and establish your research priority;
- Protect your idea from being stolen with this time-stamped preprint article;
- Enhance the exposure and impact of your research;
- Receive feedback from your peers in advance;
- Have it indexed in Web of Science (Preprint Citation Index), Google Scholar, Crossref, SHARE, PrePubMed, Scilit and Europe PMC.