Topic Editors
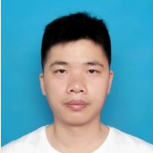
Biomarkers and Therapeutic Targets Based on Bioinformatical Studies
Topic Information
Dear Colleagues,
Bioinformatics is a rapidly evolving field that has revolutionized our understanding of biological systems and their functions. The ability to analyze large volumes of data and thereby extract meaningful information has led to the identification of biomarkers that can be used for the diagnosis and treatment of diseases. This has greatly improved patient outcomes and paved the way for personalized medicine. The integration of cutting-edge technologies into bioinformatics has greatly facilitated the identification of biomarkers and new drugs, the development of diagnostic models, the design of targeted drugs, and the personalized treatment of diseases. In the development of drug targets, bioinformatics can also be used to discover new targets, predict drug actions, evaluate drug efficacy and safety, etc. Among these tasks, drug target prediction based on bioinformatics is an incredibly important research area. By analyzing protein sequences, structures, and functions through molecular docking and molecular simulation, the interactions between drugs and proteins can be predicted, and molecules with potential drug activity can be screened. In addition, bioinformatics can also be used for the simulation and design of drug molecules, thereby optimizing the properties and effects of drugs. In summary, bioinformatics plays an important role in the development of drug targets, providing new ideas and methods for drug development.
The implementation of cutting-edge technologies such as machine learning, RNA-seq, epigenomics, and metabolomics has greatly improved our understanding of biological systems and their functions. These technologies have been used to identify biomarkers for diagnosis and treatment, develop prognostic models that can predict the outcomes of disease progression, and identify patients at high risk of developing complications. The integration of these technologies with clinical data has led to the development of personalized medicine, which has greatly improved patient outcomes and has the potential to revolutionize healthcare.
We welcome original research, reviews, and other articles relevant to the relevant Topics. Topics of interest include but are not limited to the following:
The identification of biomarkers using advanced sequencing technologies;
the development of prognostic and diagnostic models using machine learning and deep learning algorithms;
the design of targeted drugs using omics data;
the validation of biomarkers using clinical samples;
the application of advanced technologies in personalized medicine;
the integration of different omics data for a comprehensive understanding of diseases.
Dr. Qingjia Chi
Prof. Dr. Xiangcheng Chen
Topic Editors
Keywords
Participating Journals
Journal Name | Impact Factor | CiteScore | Launched Year | First Decision (median) | APC | |
---|---|---|---|---|---|---|
![]()
Bioengineering
|
4.6 | 4.2 | 2014 | 17.7 Days | CHF 2700 | Submit |
![]()
Biology
|
4.2 | 4.0 | 2012 | 18.7 Days | CHF 2700 | Submit |
![]()
Biomimetics
|
4.5 | 4.5 | 2016 | 17.2 Days | CHF 2200 | Submit |
![]()
Life
|
3.2 | 2.7 | 2011 | 17.5 Days | CHF 2600 | Submit |
![]()
Molecules
|
4.6 | 6.7 | 1996 | 14.6 Days | CHF 2700 | Submit |
MDPI Topics is cooperating with Preprints.org and has built a direct connection between MDPI journals and Preprints.org. Authors are encouraged to enjoy the benefits by posting a preprint at Preprints.org prior to publication:
- Immediately share your ideas ahead of publication and establish your research priority;
- Protect your idea from being stolen with this time-stamped preprint article;
- Enhance the exposure and impact of your research;
- Receive feedback from your peers in advance;
- Have it indexed in Web of Science (Preprint Citation Index), Google Scholar, Crossref, SHARE, PrePubMed, Scilit and Europe PMC.