Statistical Inference from High Dimensional Data II
A special issue of Entropy (ISSN 1099-4300). This special issue belongs to the section "Statistical Physics".
Deadline for manuscript submissions: closed (31 December 2021) | Viewed by 7903
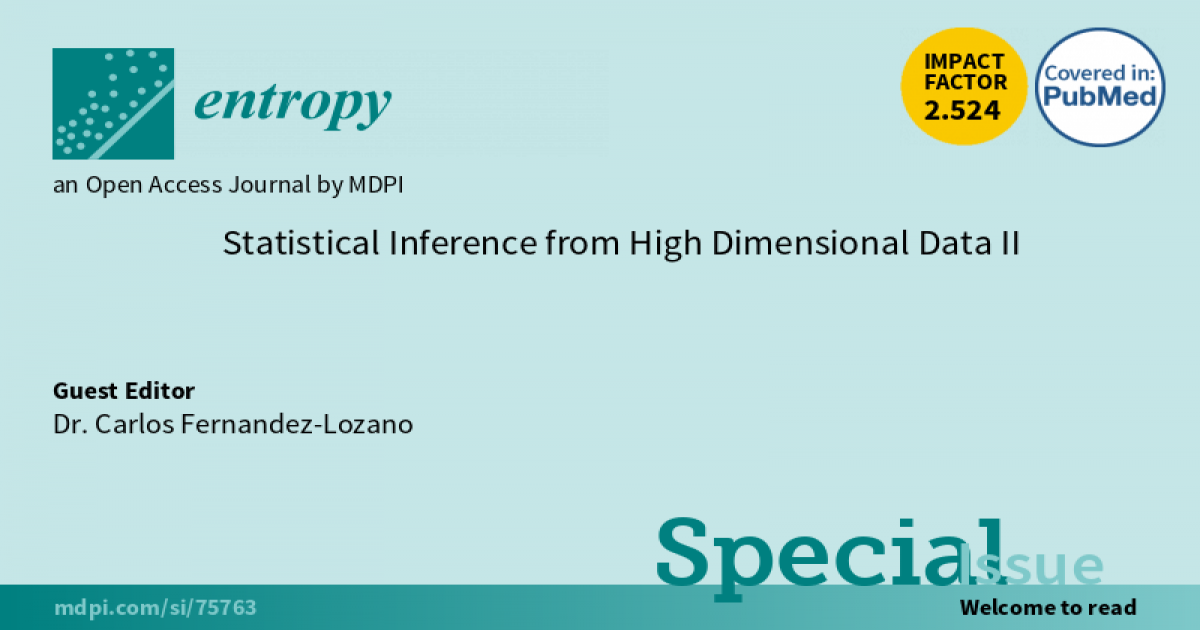
Special Issue Editor
Interests: machine learning; feature selection; complex biological systems; cancer systems; bionformatics; biomedical data science; computational biology
Special Issues, Collections and Topics in MDPI journals
Special Issue Information
Dear Colleagues,
Continuous improvement and cost reduction in next-generation sequencing platforms is enabling better understanding of multifactorial and complex pathologies such as cancer. This is the typical problem in which the amount of data matters and where, in addition, the so-called curse of dimensionality occurs (the number of variables is many orders of magnitude greater than the number of cases). In this Special Issue, we welcome contributions that apply different approaches of Statistical Inference or Machine Learning for the characterization of complex pathologies using -omic data. We strongly encourage interdisciplinary works with real data (TCGA, HMP, clinicogenomic data or related datasets) and heterogeneous data integration (clinical, genomic, proteomic, and so on).
This Special Issue solicit submissions in, but not limited to, the following areas:
- Applications based on statistical inference from high dimensional data;
- Dimensionality reduction with imbalanced biological datasets;
- Applications based on feature selection (e.g., text processing, bioinformatics, medical informatics and natural language processing);
- Applications based on Information Theory for data integration (e.g., semantic interoperability, clustering, classification);
- Applications based on feature selection methods using meta-heuristic search methods such as genetic algorithms, particle swarm optimization and so on;
- Applications based on feature extraction (e.g., PCA, LDA);
- Applications based on prior knowledge (e.g., ontologies, pathways).
Volume I: Special Issue "Statistical Inference from High Dimensional Data"
Dr. Carlos Fernandez-Lozano
Guest Editor
Manuscript Submission Information
Manuscripts should be submitted online at www.mdpi.com by registering and logging in to this website. Once you are registered, click here to go to the submission form. Manuscripts can be submitted until the deadline. All submissions that pass pre-check are peer-reviewed. Accepted papers will be published continuously in the journal (as soon as accepted) and will be listed together on the special issue website. Research articles, review articles as well as short communications are invited. For planned papers, a title and short abstract (about 100 words) can be sent to the Editorial Office for announcement on this website.
Submitted manuscripts should not have been published previously, nor be under consideration for publication elsewhere (except conference proceedings papers). All manuscripts are thoroughly refereed through a single-blind peer-review process. A guide for authors and other relevant information for submission of manuscripts is available on the Instructions for Authors page. Entropy is an international peer-reviewed open access monthly journal published by MDPI.
Please visit the Instructions for Authors page before submitting a manuscript. The Article Processing Charge (APC) for publication in this open access journal is 2600 CHF (Swiss Francs). Submitted papers should be well formatted and use good English. Authors may use MDPI's English editing service prior to publication or during author revisions.
Keywords
- Feature selection
- Machine learning
- Statistical inference
- Dimensionality
- Complex biological systems
- Multifactorial diseases
- Computational biology
- Bioinformatics
- Information theory
- Large-scale data analysis
- Information theory
- Data mining