Advanced Sensing Techniques for Intelligent Human Activity Recognition Using Machine Learning
A special issue of Electronics (ISSN 2079-9292). This special issue belongs to the section "Industrial Electronics".
Deadline for manuscript submissions: closed (28 February 2022) | Viewed by 36739
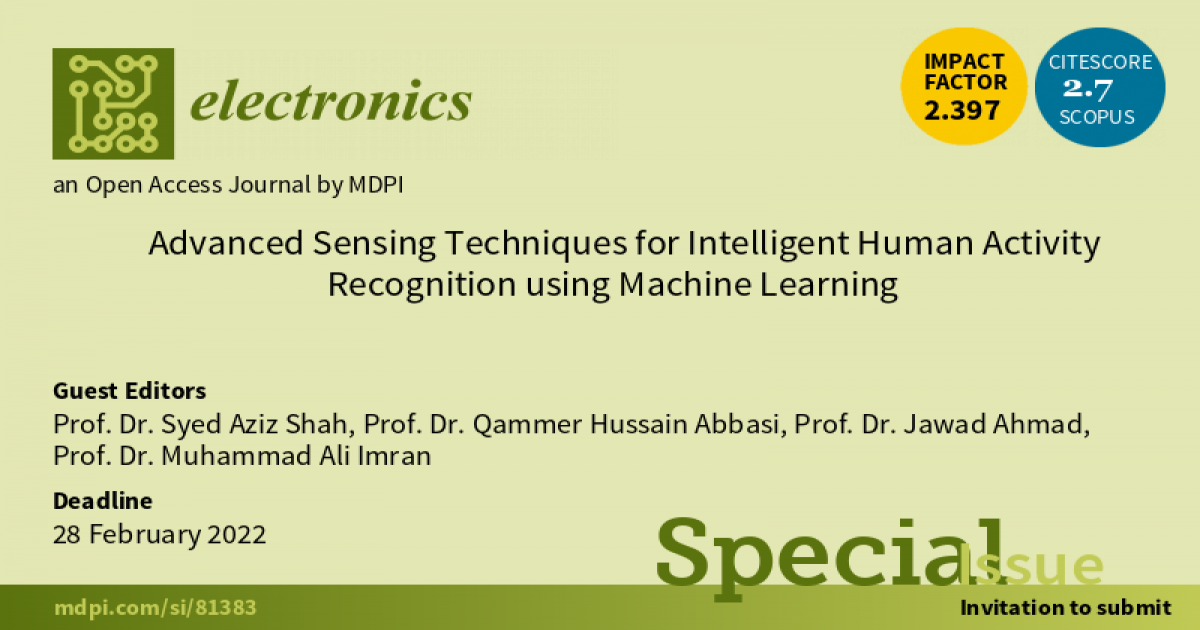
Special Issue Editors
Interests: machine learning and wireless sensing for human activity recognition; radar technology; software-defined radios; antennas and propagation; intelligent healthcare; disease monitoring; agriculture technologies; antenna interaction with the human body; wireless body sensor networks; non-invasive health care solutions
Special Issues, Collections and Topics in MDPI journals
Interests: nano communication; biomedical applications of millimeter and terahertz communication; wearable and flexible sensors; compact antenna design; RF design and radio propagation; antenna interaction with human body; implants; body centric wireless communication issues; wireless body sensor networks; non-invasive health care solutions; physical layer security for wearable/implant communication and multiple-input–multiple-output systems
Special Issues, Collections and Topics in MDPI journals
Interests: cryptography; blockchain; chaos theory; image encryption; IoT; machine learning
Special Issues, Collections and Topics in MDPI journals
Interests: 5G and Beyond networks
Special Issues, Collections and Topics in MDPI journals
Special Issue Information
Dear Colleagues,
This Special Issue invites original scientific and research articles on state-of-the-art human activity recognition, vital sign monitoring using various sensing techniques (contact and non-contact), and remote patient monitoring in the advanced intelligent healthcare sector. Additionally, this issue invites articles/reviews on cybersecurity for human activity recognition. The core idea is to provide an opportunity to academics, researchers, and industry professionals to showcase their current developments and set future directions.
The topics of interest include but are not limited to:
- Data analytics for human activity recognition in intelligent healthcare;
- Wearable sensors, devices, or techniques for physiological monitoring;
- Wearable sensors health applications;
- Software-defined radios for vital signs monitoring;
- Disease detection based on handwriting using deep learning;
- Identifying disorders using eye movements;
- Heartrate monitoring techniques using machine learning;
- Gait analysis using wireless sensing;
- Cybersecurity in remote patient monitoring.
Prof. Dr. Syed Aziz Shah
Prof. Dr. Qammer Hussain Abbasi
Prof. Dr. Jawad Ahmad
Prof. Dr. Muhammad Ali Imran
Guest Editors
Manuscript Submission Information
Manuscripts should be submitted online at www.mdpi.com by registering and logging in to this website. Once you are registered, click here to go to the submission form. Manuscripts can be submitted until the deadline. All submissions that pass pre-check are peer-reviewed. Accepted papers will be published continuously in the journal (as soon as accepted) and will be listed together on the special issue website. Research articles, review articles as well as short communications are invited. For planned papers, a title and short abstract (about 100 words) can be sent to the Editorial Office for announcement on this website.
Submitted manuscripts should not have been published previously, nor be under consideration for publication elsewhere (except conference proceedings papers). All manuscripts are thoroughly refereed through a single-blind peer-review process. A guide for authors and other relevant information for submission of manuscripts is available on the Instructions for Authors page. Electronics is an international peer-reviewed open access semimonthly journal published by MDPI.
Please visit the Instructions for Authors page before submitting a manuscript. The Article Processing Charge (APC) for publication in this open access journal is 2400 CHF (Swiss Francs). Submitted papers should be well formatted and use good English. Authors may use MDPI's English editing service prior to publication or during author revisions.