Causal and Explainable Artificial Intelligence
A special issue of Applied Sciences (ISSN 2076-3417). This special issue belongs to the section "Computing and Artificial Intelligence".
Deadline for manuscript submissions: 30 April 2024 | Viewed by 2914
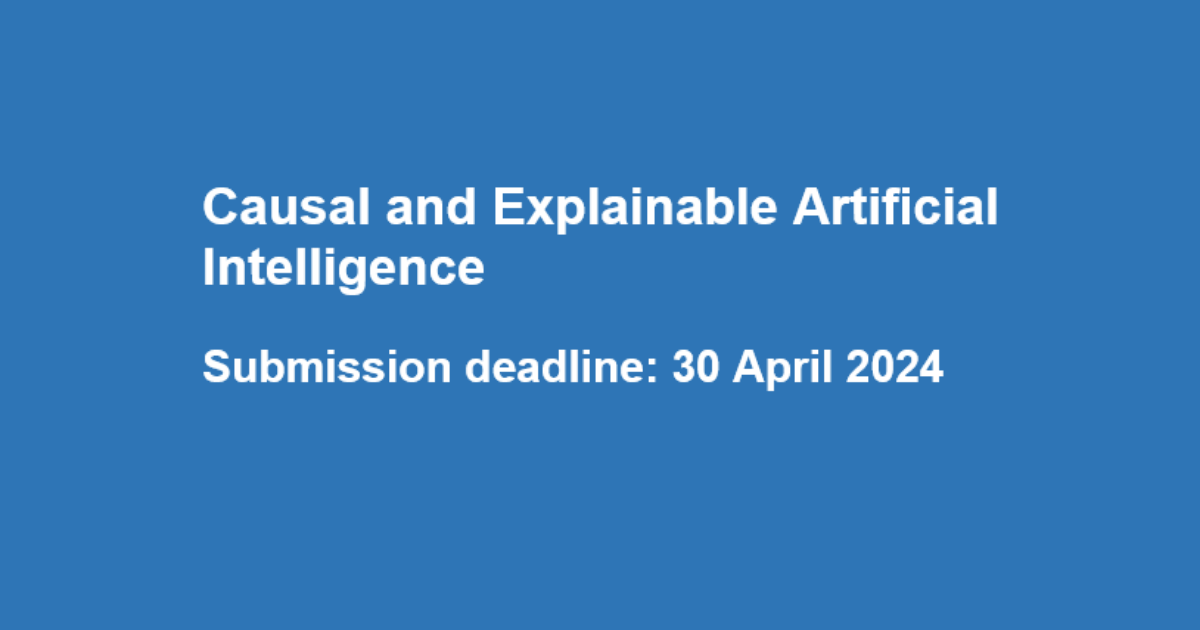
Special Issue Editors
Interests: interpretable and explainable AI; causal discovery and inference
Special Issue Information
Dear Colleagues,
Over the last decade, machine learning (ML) and artificial intelligence (AI) have been increasingly adopted in various domains. However, the lack of transparency and interpretability in AI/ML models has resulted in a growing demand to make them more understandable to humans. This is crucial for ensuring effective collaboration between humans and AI systems and for ensuring regulatory compliance.
Based on the Causal and Explainable AI (CXAI 2023) workshop, this Special Issue will present a collection of cutting-edge research and recent real-world applications in causal inference/discovery and interpretable/explainable AI, with the aim of making complex or black-box AI/ML models understandable and supporting reliable, trustable and responsible decision making.
Authors are encouraged to submit their papers to the CXAI 2023 workshop first and, if accepted, then submit extended versions of their papers to this Special Issue. Alternatively, authors may submit their papers directly to the Special Issue, without submitting to the CXAI workshop.
Dr. Yanchang Zhao
Dr. Yun-Sing Koh
Guest Editors
Manuscript Submission Information
Manuscripts should be submitted online at www.mdpi.com by registering and logging in to this website. Once you are registered, click here to go to the submission form. Manuscripts can be submitted until the deadline. All submissions that pass pre-check are peer-reviewed. Accepted papers will be published continuously in the journal (as soon as accepted) and will be listed together on the special issue website. Research articles, review articles as well as short communications are invited. For planned papers, a title and short abstract (about 100 words) can be sent to the Editorial Office for announcement on this website.
Submitted manuscripts should not have been published previously, nor be under consideration for publication elsewhere (except conference proceedings papers). All manuscripts are thoroughly refereed through a single-blind peer-review process. A guide for authors and other relevant information for submission of manuscripts is available on the Instructions for Authors page. Applied Sciences is an international peer-reviewed open access semimonthly journal published by MDPI.
Please visit the Instructions for Authors page before submitting a manuscript. The Article Processing Charge (APC) for publication in this open access journal is 2400 CHF (Swiss Francs). Submitted papers should be well formatted and use good English. Authors may use MDPI's English editing service prior to publication or during author revisions.
Keywords
- interpretable machine learning
- explainable artificial intelligence
- causal discovery
- causal inference
- counterfactual analysis