Recent Applications of Machine Learning in Natural Language Processing (NLP)
A special issue of Applied Sciences (ISSN 2076-3417). This special issue belongs to the section "Computing and Artificial Intelligence".
Deadline for manuscript submissions: 10 August 2024 | Viewed by 1121
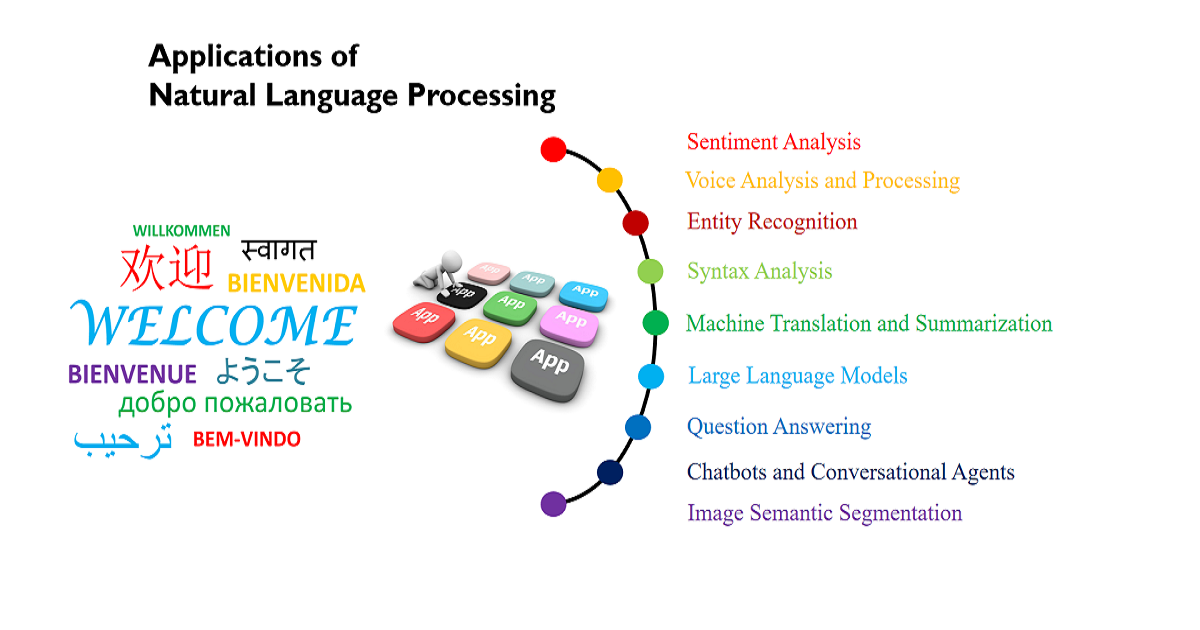
Special Issue Editors
Interests: multiagent systems; social computing; linked data; natural language processing; affect technology; machine learning
Special Issues, Collections and Topics in MDPI journals
Interests: deep learning; natural language processing; neural machine translation; corpus construction and data augmentation
Special Issue Information
Dear Colleagues,
The vibrant era of digital information has experienced a paradigm shift with the integration of machine learning in the domain of Natural Language Processing (NLP). The surge in data-driven decision making and human-like interface applications underscores the prominence and ubiquity of these technologies. This Special Issue seeks to delve deep into the recent applications of machine learning within the realm of NLP, elucidating its transformative potential in reshaping the human–computer interaction paradigm.
NLP, at its core, encompasses a spectrum of tasks ranging from sentiment analysis, which discerns underlying emotions from textual data, to voice analysis and processing, enhancing auditory interactions. The granular identification capabilities of entity recognition play a pivotal role in information retrieval, while syntax analysis helps in understanding the structural intricacies of language. Furthermore, we are witnessing revolutionary changes in the domain of machine translation and summarization, making cross-cultural and multilingual interactions seamless. Large Language Models, with their massive information processing capabilities, are augmenting question-answering systems, chatbots, and conversational agents, facilitating more organic and intuitive interactions. Additionally, the confluence of image semantic segmentation with NLP has opened avenues for more comprehensive multimedia understanding.
Relevant areas of exploration within this amalgamation of machine learning and NLP include, but are not restricted to:
- Sentiment Analysis;
- Voice Analysis and Processing;
- Entity Recognition;
- Syntax Analysis;
- Machine Translation and Summarization;
- Large Language Models;
- Question Answering;
- Chatbots and Conversational Agents;
- Image Semantic Segmentation.
In the spirit of knowledge dissemination and fostering innovation, this Special Issue aims to amass high-quality, original research papers that elucidate the recent applications, challenges, and future prospects of machine learning in NLP.
Dr. Carlos A. Iglesias
Guest Editor
Dr. Jinyi Zhang
Guest Editor Assistant
Manuscript Submission Information
Manuscripts should be submitted online at www.mdpi.com by registering and logging in to this website. Once you are registered, click here to go to the submission form. Manuscripts can be submitted until the deadline. All submissions that pass pre-check are peer-reviewed. Accepted papers will be published continuously in the journal (as soon as accepted) and will be listed together on the special issue website. Research articles, review articles as well as short communications are invited. For planned papers, a title and short abstract (about 100 words) can be sent to the Editorial Office for announcement on this website.
Submitted manuscripts should not have been published previously, nor be under consideration for publication elsewhere (except conference proceedings papers). All manuscripts are thoroughly refereed through a single-blind peer-review process. A guide for authors and other relevant information for submission of manuscripts is available on the Instructions for Authors page. Applied Sciences is an international peer-reviewed open access semimonthly journal published by MDPI.
Please visit the Instructions for Authors page before submitting a manuscript. The Article Processing Charge (APC) for publication in this open access journal is 2400 CHF (Swiss Francs). Submitted papers should be well formatted and use good English. Authors may use MDPI's English editing service prior to publication or during author revisions.
Keywords
- sentiment analysis
- voice analysis and processing
- entity recognition
- syntax analysis
- machine translation and summarization
- large language models
- question answering
- chatbots and conversational agents
- image semantic segmentation