Internet of Things: Recent Advances and Applications
A special issue of Applied Sciences (ISSN 2076-3417). This special issue belongs to the section "Computing and Artificial Intelligence".
Deadline for manuscript submissions: 30 September 2024 | Viewed by 972
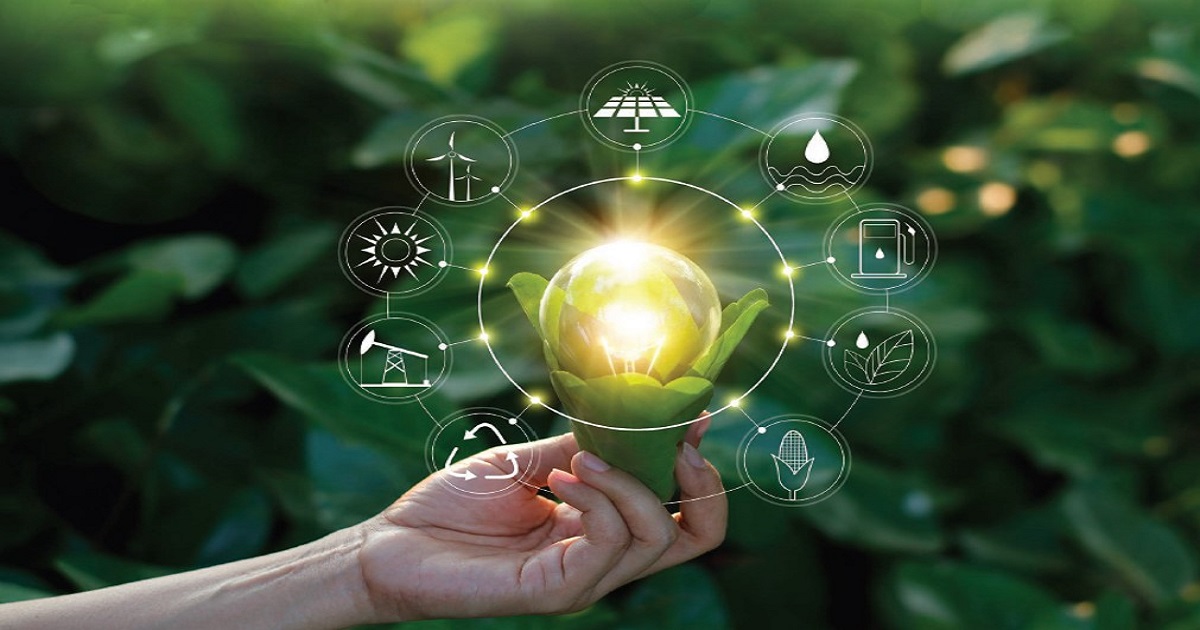
Special Issue Editors
Interests: adversarial machine learning; data poisoning and defense; network security; Internet of Things
Interests: data security; blockchain; privacy computing; intelligent Internet of Things
Interests: cyberspace security; information security; e-government collaborative work and secure data exchange theory and application technology; content security management theory and application
Special Issue Information
Dear Colleagues,
The Internet of Things (IoT) could provide ubiquitous low-latency connectivity for various new applications such as the metaverse, industry 5.0, and space–ground integration via large-scale models, edge AI, blockchain technology, coded computing, etc.
With the emergence of new technologies, the field of IoT has witnessed significant development in recent years, especially in terms of data processing, context awareness, coverage optimization, energy consumption control, and security protection.
This Special Issue aims to collate high-quality and original works regarding the recent advances and applications of Internet of Things technology. Potential topics include (but are not limited to) the following:
- IoT massive data sharing and processing via edge AI;
- IoT sensing and networking via semantic communication;
- IoT energy saving via advanced low-power designs;
- IoT coverage optimization via 5G/6G networking;
- IoT interoperability via large-scale models;
- IoT malware detection via deep learning;
- IoT vulnerability identification;
- IoT data poisoning and defense;
- IoT security and privacy via blockchain technology;
- Underwater IoT applications;
- IoT applications in space;
- Experimental IoT prototyping and testbeds.
Dr. Gaolei Li
Dr. Xi Lin
Prof. Dr. Jianhua Li
Guest Editors
Manuscript Submission Information
Manuscripts should be submitted online at www.mdpi.com by registering and logging in to this website. Once you are registered, click here to go to the submission form. Manuscripts can be submitted until the deadline. All submissions that pass pre-check are peer-reviewed. Accepted papers will be published continuously in the journal (as soon as accepted) and will be listed together on the special issue website. Research articles, review articles as well as short communications are invited. For planned papers, a title and short abstract (about 100 words) can be sent to the Editorial Office for announcement on this website.
Submitted manuscripts should not have been published previously, nor be under consideration for publication elsewhere (except conference proceedings papers). All manuscripts are thoroughly refereed through a single-blind peer-review process. A guide for authors and other relevant information for submission of manuscripts is available on the Instructions for Authors page. Applied Sciences is an international peer-reviewed open access semimonthly journal published by MDPI.
Please visit the Instructions for Authors page before submitting a manuscript. The Article Processing Charge (APC) for publication in this open access journal is 2400 CHF (Swiss Francs). Submitted papers should be well formatted and use good English. Authors may use MDPI's English editing service prior to publication or during author revisions.
Keywords
- Internet of Things
- large-scale model
- blockchain
- edge AI
- security and privacy