Machine Learning in Audio Signal Processing and Music Information Retrieval
A special issue of Applied Sciences (ISSN 2076-3417). This special issue belongs to the section "Computing and Artificial Intelligence".
Deadline for manuscript submissions: 20 June 2024 | Viewed by 4055
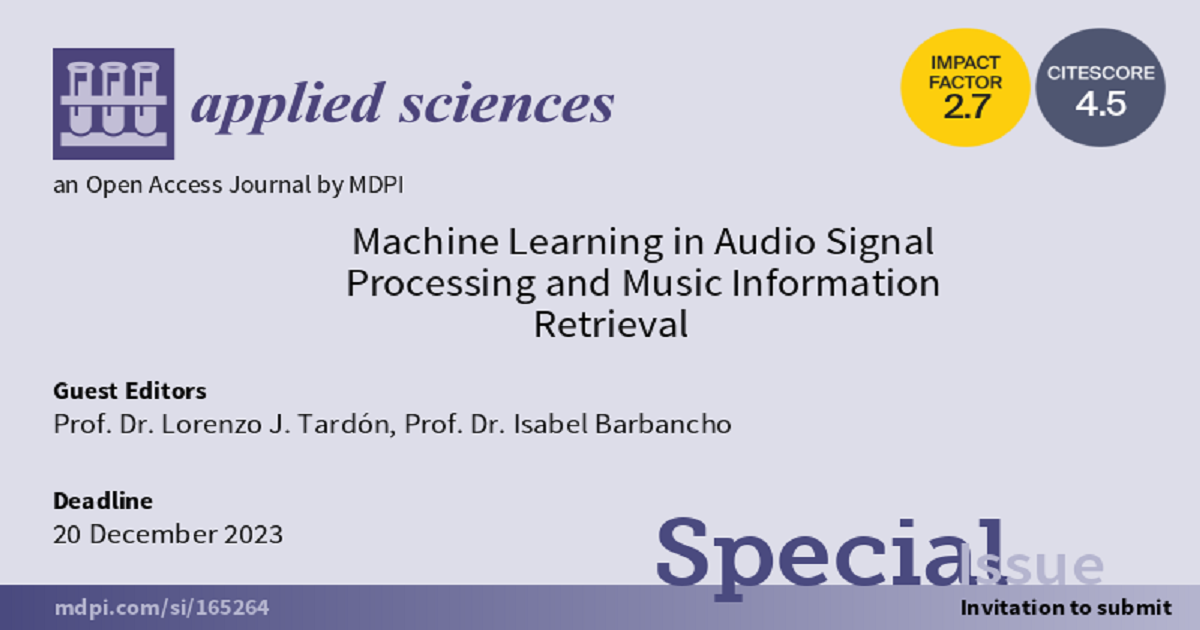
Special Issue Editors
Interests: serious games; digital audio and image processing; pattern analysis and recognition and applications of signal processing techniques and methods
Special Issues, Collections and Topics in MDPI journals
Interests: music information retrieval; audio signal processing; machine learning; musical acoustics; serious games; eeg signal processing; multimedia aplications
Special Issues, Collections and Topics in MDPI journals
Special Issue Information
Dear Colleagues,
Machine learning methods and applications have been utilized for a while; recently, there has been a growth in the multimedia content and databases available, as well as computational advances, artificial intelligence techniques, and, especially, deep learning methods. These have spread across all application areas in the multimedia signal application framework and, within this context, in the audio and music signal research topics, including music information retrieval.
These methods cover a wide range of techniques, from classical machine learning methods to the recently developed deep neural networks, with an application in a large variety of tasks including audio classification, source separation, enhancement, transcription, indexation, content creation, entertainment, gaming, etc.
In this context, there is still ample room for research in innovation. This Special Issue aims to provide the research community with a space to share their recent findings and advances. The topics of interest include, but are not limited to, the following:
- Machine learning methods for music/audio information retrieval, indexation, and querying;
- Music instrument identification, synthesis, transformation, and classification;
- Symbolic music processing;
- Machine learning for the discovery of musical structure, segmentation, and form: melody and motives, harmony, chords and tonality, rhythm, beat, tempo, timbre, instrumentation and voice, style, and genre;
- Musical content creation: melodies, accompaniment, orchestration, etc;
- Machine learning methods for natural language processing, text, and web mining;
- Sound source separation;
- Music transcription and annotation, alignment, synchronization, and score following. Optical music recognition;
- Audio fingerprinting;
- Machine learning approaches for visualization, auralization, and sonification;
- Music recommendation and playlist generation;
- Music and health, wellbeing, therapy, music training, and education;
- Machine learning methods for music and audio in gaming.
Prof. Dr. Lorenzo J. Tardón
Prof. Dr. Isabel Barbancho
Guest Editors
Manuscript Submission Information
Manuscripts should be submitted online at www.mdpi.com by registering and logging in to this website. Once you are registered, click here to go to the submission form. Manuscripts can be submitted until the deadline. All submissions that pass pre-check are peer-reviewed. Accepted papers will be published continuously in the journal (as soon as accepted) and will be listed together on the special issue website. Research articles, review articles as well as short communications are invited. For planned papers, a title and short abstract (about 100 words) can be sent to the Editorial Office for announcement on this website.
Submitted manuscripts should not have been published previously, nor be under consideration for publication elsewhere (except conference proceedings papers). All manuscripts are thoroughly refereed through a single-blind peer-review process. A guide for authors and other relevant information for submission of manuscripts is available on the Instructions for Authors page. Applied Sciences is an international peer-reviewed open access semimonthly journal published by MDPI.
Please visit the Instructions for Authors page before submitting a manuscript. The Article Processing Charge (APC) for publication in this open access journal is 2400 CHF (Swiss Francs). Submitted papers should be well formatted and use good English. Authors may use MDPI's English editing service prior to publication or during author revisions.
Keywords
- music information retrieval
- machine learning for audio and music
- intelligent audio signal processing
- audio analysis and transformation