Unveiling Open World Challenges: Strengthening Model Adaptability beyond Training Data
A special issue of Applied Sciences (ISSN 2076-3417). This special issue belongs to the section "Computing and Artificial Intelligence".
Deadline for manuscript submissions: 31 August 2024 | Viewed by 805
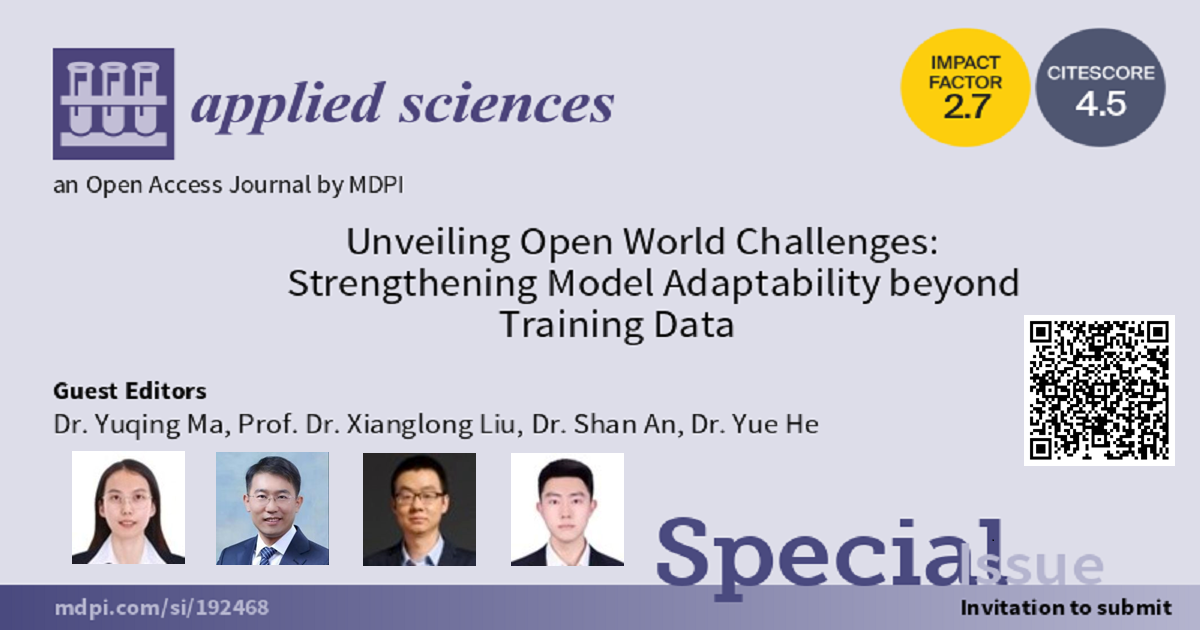
Special Issue Editors
Interests: open-world learning; few-shot learning; meta-learning; generative adversarial network
Interests: fast visual computing (e.g., large-scale search/understanding) and robust deep learning (e.g., network quantization, adversarial attack/defense, few shot learning)
Special Issues, Collections and Topics in MDPI journals
Interests: image segmentation; extended reality; robotics; 3D reconstruction
Special Issue Information
Dear Colleagues,
We are inviting submissions for the Special Issue on “Unveiling Open World Challenges: Enhancing Model Reliability Beyond Training Data”.
While machine learning and AI have made significant strides, models often struggle to capture the complexity of the real world with limited training data. This Issue aims to address the disparity between training and real-world scenarios, emphasizing the hurdles AI encounters in adapting to unforeseen circumstances. Our goal is to align AI capabilities more closely with human adaptability, which is essential for navigating unpredictable real-world applications.
This timely topic has garnered significant attention for its practical implications. We invite contributors to share innovative ideas and profound insights capable of revolutionizing the generalization capabilities of AI models, ensuring their reliability and consistent performance within the open world. This endeavor aims to bolster the progression of AI applications in real-world settings.
In this Special Issue, we encourage submissions from diverse fields including open-world learning, few/zero-shot learning, domain adaptation, artificial intelligence alignment, uncertainty quantification, and risk assessment methods, among others. We seek to explore pioneering methodologies and approaches to align AI models, welcoming both theoretical and empirical studies, comprehensive reviews, and surveys.
We eagerly anticipate your contributions to this discourse.
Dr. Yuqing Ma
Prof. Dr. Xianglong Liu
Dr. Shan An
Dr. Yue He
Guest Editors
Manuscript Submission Information
Manuscripts should be submitted online at www.mdpi.com by registering and logging in to this website. Once you are registered, click here to go to the submission form. Manuscripts can be submitted until the deadline. All submissions that pass pre-check are peer-reviewed. Accepted papers will be published continuously in the journal (as soon as accepted) and will be listed together on the special issue website. Research articles, review articles as well as short communications are invited. For planned papers, a title and short abstract (about 100 words) can be sent to the Editorial Office for announcement on this website.
Submitted manuscripts should not have been published previously, nor be under consideration for publication elsewhere (except conference proceedings papers). All manuscripts are thoroughly refereed through a single-blind peer-review process. A guide for authors and other relevant information for submission of manuscripts is available on the Instructions for Authors page. Applied Sciences is an international peer-reviewed open access semimonthly journal published by MDPI.
Please visit the Instructions for Authors page before submitting a manuscript. The Article Processing Charge (APC) for publication in this open access journal is 2400 CHF (Swiss Francs). Submitted papers should be well formatted and use good English. Authors may use MDPI's English editing service prior to publication or during author revisions.
Keywords
- open set/world learning problem
- few-/zero-shot learning
- evaluating model generalization
- transfer learning
- physics-informed learning
- adaptive artificial intelligence algorithms
- out-of-distribution generalization
- quantification of uncertainty and risk