Predictive Analytics and Fault Diagnosis of Machines with Machine Learning Techniques
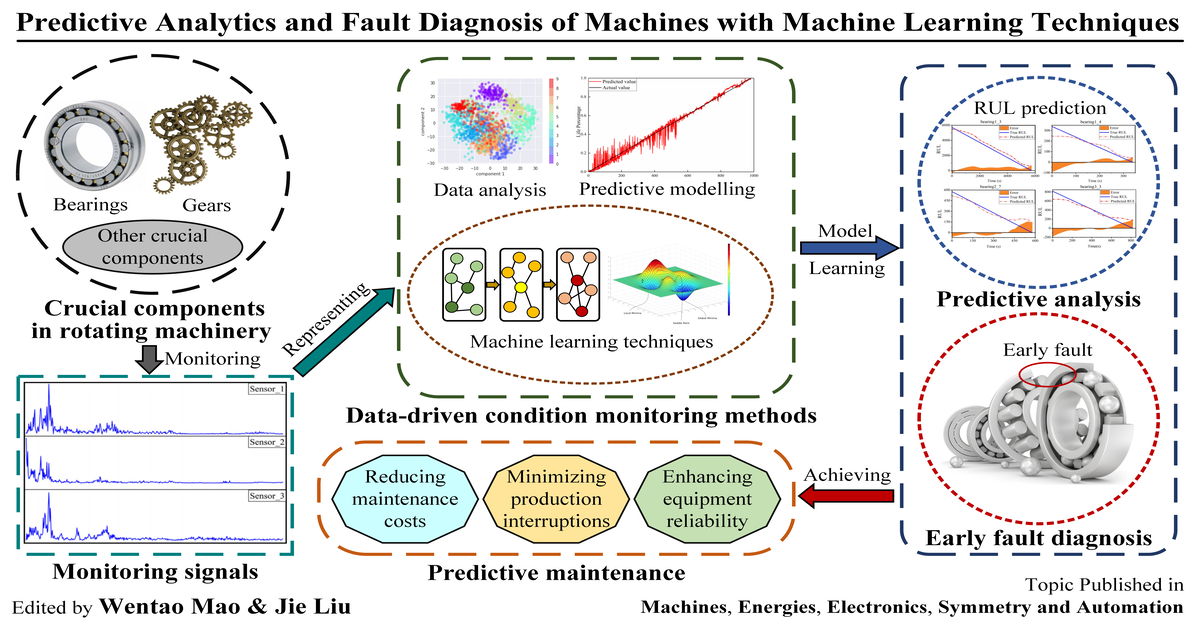
Topic Information
Dear Colleagues,
This Topic focuses on cutting-edge topics in the industrial sector, exploring how predictive analytics and machine fault diagnosis can enhance production efficiency and equipment reliability. With the emergence of Industry 4.0, data-driven decision-making and innovative maintenance strategies have become the cornerstone of the industry. This Topic delves into the methods of employing data analysis, predictive modelling, and machine learning techniques to achieve machine health monitoring and early fault diagnosis, ultimately reducing maintenance costs, minimizing production interruptions, and enhancing equipment reliability. We pay particular attention to critical components in rotating machinery, such as bearings, which play a pivotal role in industrial manufacturing. Through state monitoring and fault diagnosis, alongside emerging technologies like deep learning, we accurately diagnose machine conditions and proactively engage in predictive maintenance. This Topic is designed to foster collaboration between industry and academia, driving innovation in methods and applications to meet the demands of modern industrial production. We eagerly anticipate research findings in this critical field, which will provide the industry with more efficient and reliable production methods.
Prof. Dr. Wentao Mao
Dr. Jie Liu
Topic Editors
Keywords
- fault diagnostics
- remaining useful life prediction
- predictive maintenance
- condition monitoring
- real-time
- machine learning
- deep learning
Participating Journals
Journal Name | Impact Factor | CiteScore | Launched Year | First Decision (median) | APC | |
---|---|---|---|---|---|---|
![]()
Automation
|
- | - | 2020 | 26.3 Days | CHF 1000 | Submit |
![]()
Electronics
|
2.9 | 4.7 | 2012 | 15.6 Days | CHF 2400 | Submit |
![]()
Energies
|
3.2 | 5.5 | 2008 | 16.1 Days | CHF 2600 | Submit |
![]()
Machines
|
2.6 | 2.1 | 2013 | 15.6 Days | CHF 2400 | Submit |
![]()
Symmetry
|
2.7 | 4.9 | 2009 | 16.2 Days | CHF 2400 | Submit |
MDPI Topics is cooperating with Preprints.org and has built a direct connection between MDPI journals and Preprints.org. Authors are encouraged to enjoy the benefits by posting a preprint at Preprints.org prior to publication:
- Immediately share your ideas ahead of publication and establish your research priority;
- Protect your idea from being stolen with this time-stamped preprint article;
- Enhance the exposure and impact of your research;
- Receive feedback from your peers in advance;
- Have it indexed in Web of Science (Preprint Citation Index), Google Scholar, Crossref, SHARE, PrePubMed, Scilit and Europe PMC.