Mobile Computing for Smart Health
A special issue of Sensors (ISSN 1424-8220). This special issue belongs to the section "Wearables".
Deadline for manuscript submissions: closed (25 December 2023) | Viewed by 4546
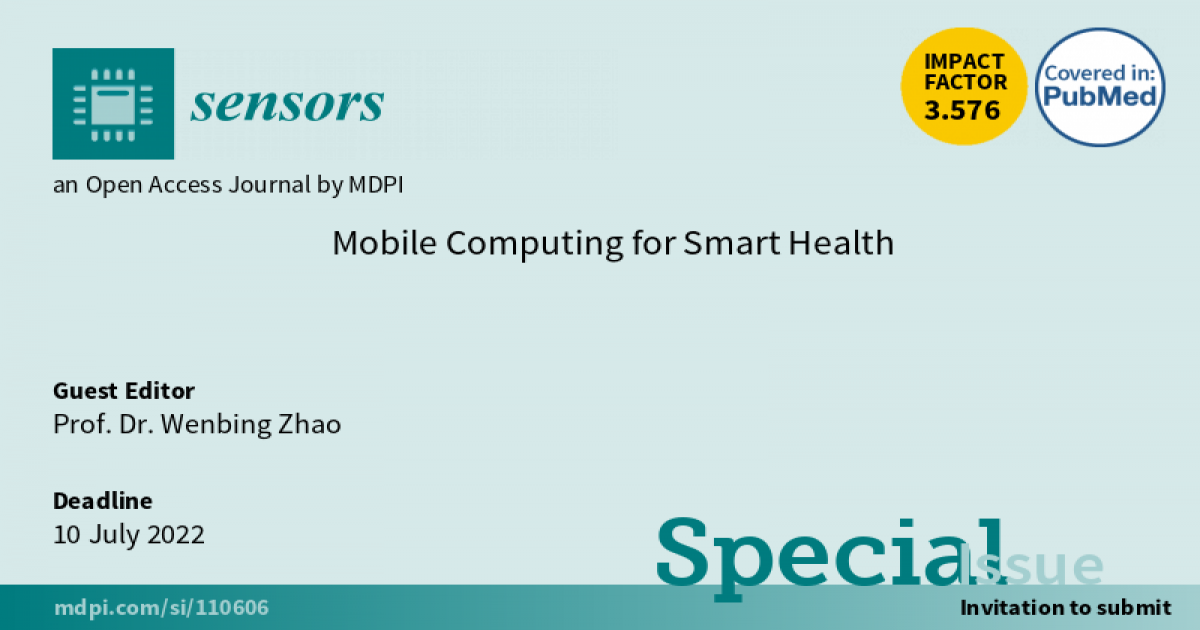
Special Issue Editor
Interests: computational intelligence; Internet of Things; big data
Special Issues, Collections and Topics in MDPI journals
Special Issue Information
Dear Colleagues,
Mobile devices, especially smartphones, are playing an essential role in people’s lives. Such devices embedded many sensors and could be used to collect information regarding the activities of daily living. Mobile devices have been proven to offer a great means to collect important information, which can help in understanding the physical and mental health status of patients and enacting intervention for patients. Mobile devices constitute an indispensable vehicle to conduct telemedicine, which is especially relevant during the prolonged pandemic. This line of research is often referred to as mHealth. The research and development in the field of mHealth is highly active with over 1000 papers published annually over the last 5 years according to Web of Science using mHealth as the only keyword. In addition to the many opportunities of applying mHealth in the understanding, diagnosis, and intervention for various physical and mental diseases, there are some fundamental gaps that deserve to be investigated. For example, it is well known that the abandon rate is high among patients who use mobile apps for intervention. Designing mobile apps that are engaging could advance the state of the art in human–computer interaction, as well as improve the efficacies of mhealth. mHealth could also incorporate other disrupt technologies, such as blockchain, to increase transparency, accountability, and integrity for clinical trials using mobile apps.
Mobile devices embedded many traditional sensors (such as an accelerometer, a gyroscope, and light sensors) as well as non-traditional sensors (such as Bluetooth LE, cameras, and microphones) to collect various information of the users of the devices. Tracking of app usage patterns could also be regarded as a form of sensing. As such, this Special Issue is highly relevant to the mission of the journal.
Prof. Dr. Wenbing Zhao
Guest Editor
Manuscript Submission Information
Manuscripts should be submitted online at www.mdpi.com by registering and logging in to this website. Once you are registered, click here to go to the submission form. Manuscripts can be submitted until the deadline. All submissions that pass pre-check are peer-reviewed. Accepted papers will be published continuously in the journal (as soon as accepted) and will be listed together on the special issue website. Research articles, review articles as well as short communications are invited. For planned papers, a title and short abstract (about 100 words) can be sent to the Editorial Office for announcement on this website.
Submitted manuscripts should not have been published previously, nor be under consideration for publication elsewhere (except conference proceedings papers). All manuscripts are thoroughly refereed through a single-blind peer-review process. A guide for authors and other relevant information for submission of manuscripts is available on the Instructions for Authors page. Sensors is an international peer-reviewed open access semimonthly journal published by MDPI.
Please visit the Instructions for Authors page before submitting a manuscript. The Article Processing Charge (APC) for publication in this open access journal is 2600 CHF (Swiss Francs). Submitted papers should be well formatted and use good English. Authors may use MDPI's English editing service prior to publication or during author revisions.