Thermal & Infrared Imaging and Sensing in Biomedical Applications
A special issue of Sensors (ISSN 1424-8220). This special issue belongs to the section "Sensing and Imaging".
Deadline for manuscript submissions: closed (31 October 2022) | Viewed by 3943
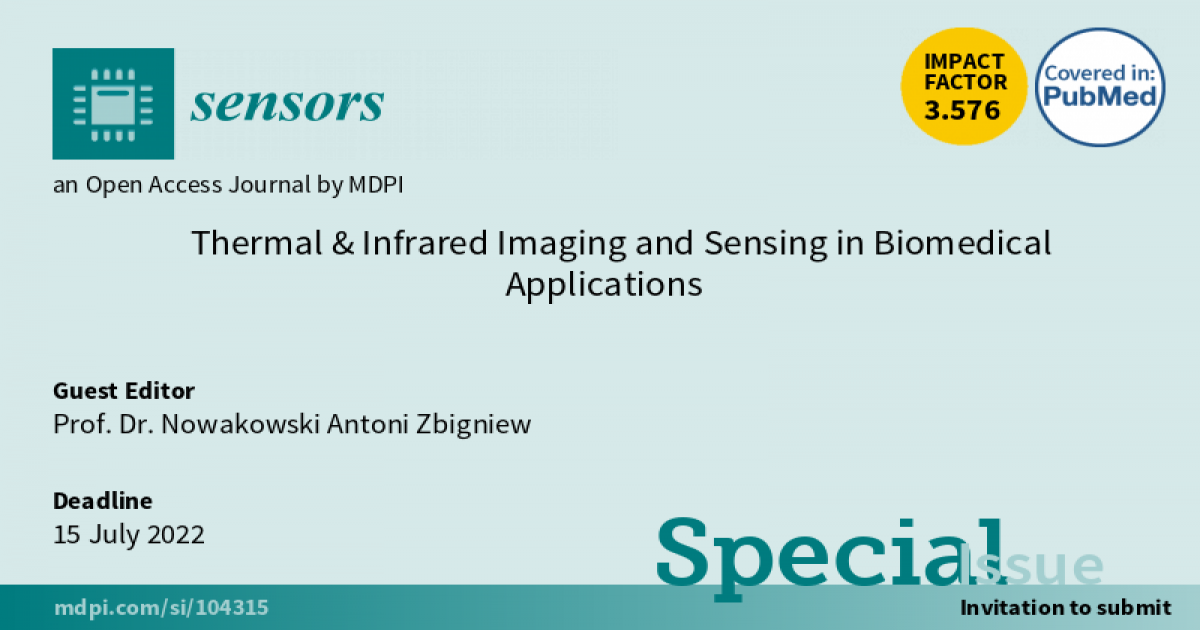
Special Issue Editor
Interests: measurement and diagnostic instrumentation & data acquisition systems; modelling and simulation in non-invasive medical diagnostics; visualization and reconstruction methods; passive and active thermography in medicine; use of informatics & telematics in research and education
Special Issues, Collections and Topics in MDPI journals
Special Issue Information
Dear Colleagues,
Recent advances of sensor technology in infrared and thermal imaging, combined with modern deep learning & Artificial Intelligence algorithms applied for interpretation of digital content, are pushing biomedical applications in diagnostics, early screening, quantitative evaluation of data, including development and evaluation of diagnostic and therapeutic procedures in medicine, veterinary, sport as well as in physiology and psychology studies. Multimodality data are key factors for improvement of quantitative description of biomedical data and improvement of diagnostic, treatment and rehabilitation quality. This special issue of Sensors is devoted to discuss and report on novel applications of sensor technology especially developed to meet requirements of biomedical expectations as well as the use of versatile commercially available instruments and systems adapted for such applications. Paper proposals describing specific applications of static and dynamic thermography in diagnostics, therapy, rehabilitation, screening, development of safety systems, biometrics etc. are welcome. New procedures, algorithms and solutions at the preclinical stage as well as results of broad clinical studies are of special appreciation. In the case of publishing proper number of high quality papers, an issue of a specialized monograph book is assumed.
Prof. Dr. Nowakowski Antoni Zbigniew
Guest Editor
If you have any questions or need further information, please free to contact Special Issue Editor Larissa Zhang <larissa.zhang@mdpi.com>.
Manuscript Submission Information
Manuscripts should be submitted online at www.mdpi.com by registering and logging in to this website. Once you are registered, click here to go to the submission form. Manuscripts can be submitted until the deadline. All submissions that pass pre-check are peer-reviewed. Accepted papers will be published continuously in the journal (as soon as accepted) and will be listed together on the special issue website. Research articles, review articles as well as short communications are invited. For planned papers, a title and short abstract (about 100 words) can be sent to the Editorial Office for announcement on this website.
Submitted manuscripts should not have been published previously, nor be under consideration for publication elsewhere (except conference proceedings papers). All manuscripts are thoroughly refereed through a single-blind peer-review process. A guide for authors and other relevant information for submission of manuscripts is available on the Instructions for Authors page. Sensors is an international peer-reviewed open access semimonthly journal published by MDPI.
Please visit the Instructions for Authors page before submitting a manuscript. The Article Processing Charge (APC) for publication in this open access journal is 2600 CHF (Swiss Francs). Submitted papers should be well formatted and use good English. Authors may use MDPI's English editing service prior to publication or during author revisions.
Keywords
- infrared & thermal imaging
- biomedical applications
- quantitative analysis
- diagnostics, therapy, rehabilitation quality
- medicine, veterinary, sport, physiology, psychology